So, for my winter break this year (2022), I didn’t travel or do very much, but I did spend some time working on a personal project.
You might be familiar with the OpenAI system, which has been getting all kinds of good press lately for its ChatGPT software, and for good reason. Their text completion system is groundbreaking, and already we are seeing a new stream of products and services to leverage it. Heck, even my holiday cards for 2022 were built using OpenAI and other systems. I’ve been finding it fascinating, and I’ve spent a fair amount of time exploring the possibilities it opens up, and that was my inspiration for this project.
The project I built is called News Mash, and it is essentially a news website which, using AI and natural language processing, automatically writes itself. You can find it online now at https://www.news-mash.com
The question is, how does it work, and what kinds of problems does it solve? Before I get into the nuts and bolts of the functionality, let’s discuss some benefits of AI-enhanced journalism.
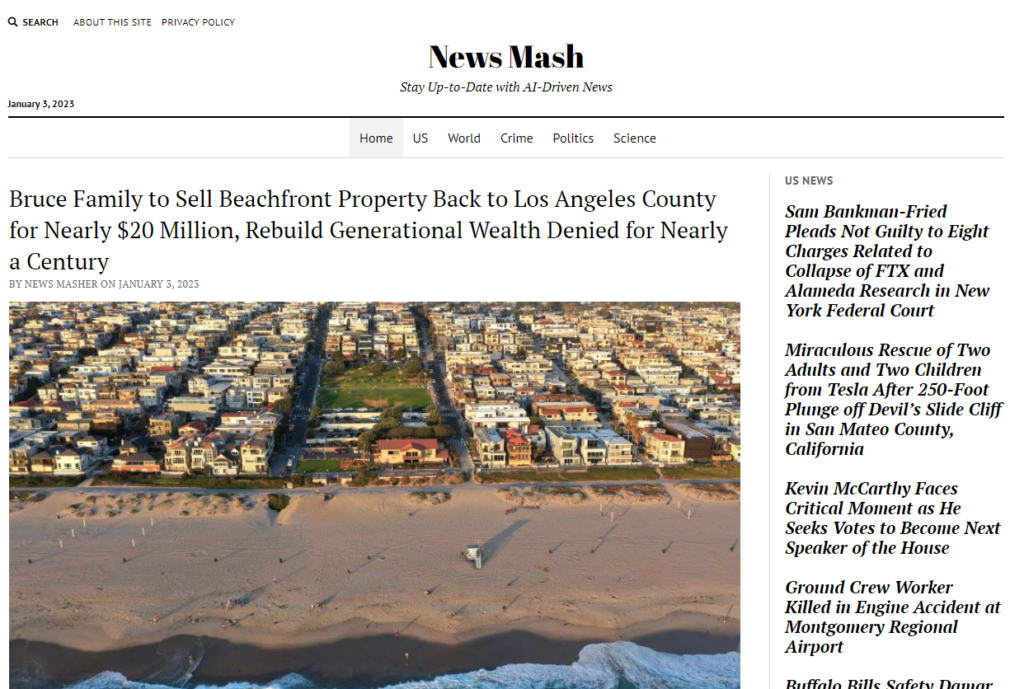
Why AI Journalism?
There are a number of problems AI-enhanced journalism addresses:
- Broader access to news and information. Many (most) reliable sources for news reporting these days are locked behind paywalls. This makes sense; journalists are humans who need to make a living from their work. The job is hard, and creating original reporting requires hard work, long hours, and a commitment to a process which very often these days ends up with the journalists being criticized, demonized and underpaid. However, there’s an argument to be made that a well-educated population is necessary for a democracy to function, and so there should be a place in the world for free information resources. For low-quality information? That’s not as much an issue: Fox News and Breitbart certainly don’t have paywalls. If they did, they’d go out of business.
- Fairness and accuracy in reporting. Bias is an inescapable reality of human communications of all kinds. There is no escape from the hardware defects embedded in all our minds. The Goldilocks effect. Confirmation bias. Political partisanship. The list goes on. However, by leveraging systems that work algorithmically instead of on feelings or instincts, and by plucking data from several sources instead of a single author, we can help mitigate some of those biases. By identifying and focusing on the key information from different content, and extracting those concept and drilling in on them, while disregarding opinion or extraneous details, News Mash lets the reader drill down to relevant information quickly.
- Minimizing editorial selection bias. No story is published by News Mash which doesn’t have at least two sources from different news organizations. Through this mechanism, News Mash eliminates advertorial and editorial content, and the articles it generates reflect the fact that only those stories which were important enough to appear in multiple outlets at around the same time are chosen for inclusion. You get only the most important news first. Eliminating advertorial and editorial content also means you don’t spend your precious attention sifting through mountains of content designed only to get you to buy something from their sponsors.
Ok, enough about the benefits. Let’s get into the details.
How does News Mash work?
TL;DR: News Mash is software written in Python which runs inside a docker container. The stories it generates are published to a WordPress website via REST API calls. Every thirty minutes or so, it runs through a loop to collect stories from various news sources. It then analyzes each story using natural language processing to figure out what the story is about, and it compares each story to all the other stories that were also published around the same time. When it finds two stories which are similar enough to conclude they are about the same subjects and events, the software combines the content of the two stories, and then, using additional NLP strategies, extracts only the most important parts of the combined text into a new summary.
This summary is then re-written into a new article (this is an important point; the stories it generates are not copies or “spun” versions of any single source, they are new, novel content). With a little more processing, we get named entities out of the new story to use as WordPress tags, and a headline is generated automatically.
Once everything is ready, News Mash posts it to a website using WordPress’ REST API. A tweet is sent out automatically via automation software in WordPress. The python application goes to sleep for half an hour, and then wakes up and does it all again.
Now that is something of an oversimplification of the actual algorithm, but it’s more or less correct. For a more detailed look at what happens, I’ve created this diagram showing a little more detail.
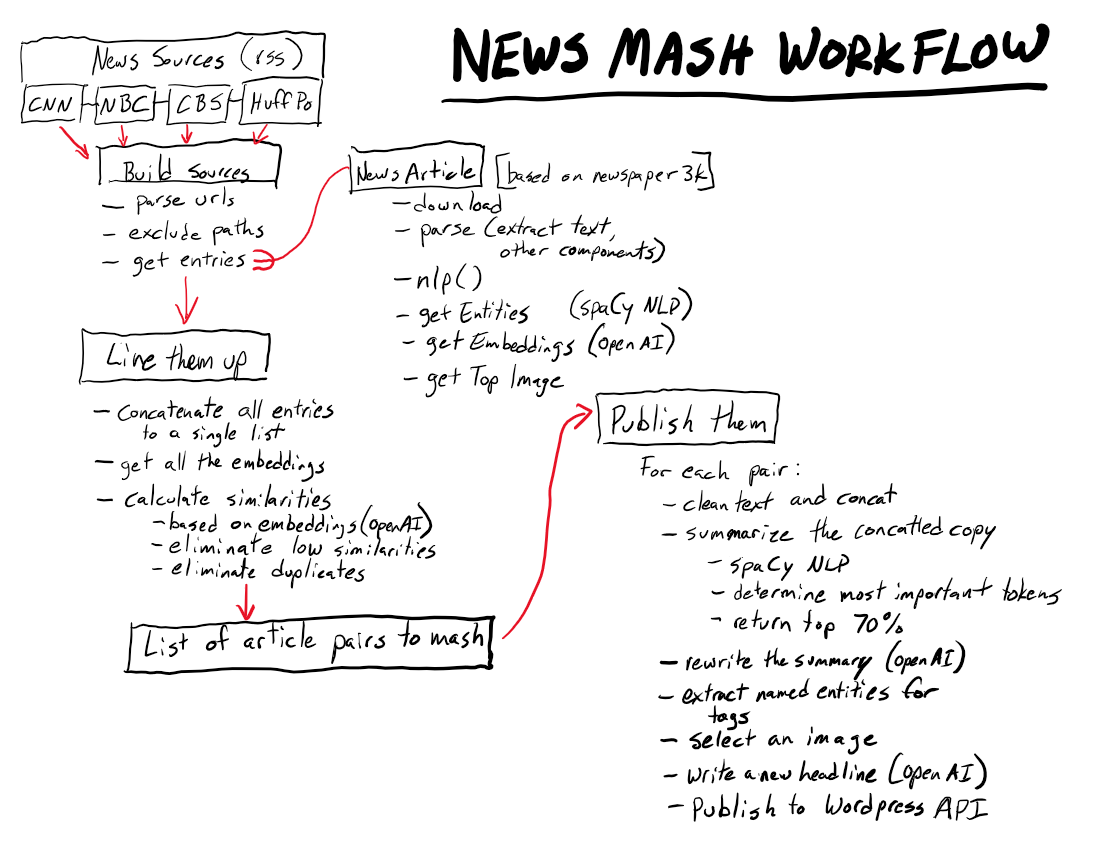
That’s kind of a lot of information, so I’ll give a little more detail on some of the more interesting steps.
How do you determine similarity between articles?
This is actually a little more tricky than you might think. You can’t just compare samples of text; you need some way of quantifying what an article is about, and you need to be able to compare it with other articles, apples-to-apples.
The way I approach this is fairly simple, and it uses two key tricks: named entity-extraction and OpenAI’s embeddings API.
First, the text of each article is run through entity extraction via the spaCy python library. That yields a list of named entities, e.g., the people, places, and things mentioned in the article. I disregard some extraneous entities (such as currencies, dates, ordinal and cardinal values, precents, quantities, etc.) since those don’t help much in this use case.
After we have a list of named entities, that list is fed into OpenAI’s embedding API, which turns it in to a vector of floating point numbers. This lets us compare the relatedness of strings. This is a super useful technique for finding clusters, anomalies, and doing a bit of classifying. Once we have a vector of embeddings for each article, we can calculate their distance from one another. The closer the vectors are geometrically, the more closely related you can consider them. We then determine the closest match for each story from the other available stories, and stick them into a list.
Of course, that list is going to include two of every pair. Story X is related to Story Y by precisely the same distance as Story Y is related to Story X, so we de-duplicate the list based on identical distance calculations.
How do you choose the most important information to include from each article?
This is fairly straightforward. Each article is tokenized, and tokens are counted up to find out which are most frequently appearing. We then select the top 70% or so of the sentences which include the most important tokens, and include only those sentences in the summary of the concatenated article texts.
This concatenated summary then becomes the grist for the next step, generating the new news article
How do you generate the new news article?
We do this using OpenAI’s completion API. In fact, this project would not be possible without the GPT-3 system that OpenAI makes available for public use. We prompt GPT-3 to take the information we have collected in the steps above, and summarize it into a new news article. The precise prompt is configurable and tunable, allowing us to make changes and adjustments based on what kind of content we are working with, and what kind of output we want to get.
We do entity extraction again on the completed new article, along with headline generation and a few other cleanup processes to make the content ready to post. And finally, we use the WordPress REST API to publish it without human intervention.
Drawbacks and shortcomings with the News Mash approach to AI-driven journalism
News Mash is not a perfect system, but it works pretty well. Here are a few of the notable shortcomings I’ve discovered thus far.
- Timeliness. The main process for News Mash takes a fair amount of time to run, currently on the order of tens of minutes, as it is relying on remote API calls and fairly intensive processing. It also relies on human reporting to know what is going on and what it should generate new articles about. Thus, it will never “break” a news story of its own. Its work is essentially derivative.
- No investigative reporting. Obviously, the system can only summarize what it finds elsewhere, it cannot go out and talk to sources, generate new leads, that sort of thing.
- Repetitiveness. I find that frequently, for big stories that have lots of coverage, the system will generate multiple articles using different sources, leading to redundancy. I think this is a problem I can solve with a little more tinkering with the algorithms though.
- Headlines can be challenging. GPT-3 has very definite ideas about how to construct headlines, which is not optimal. Again, I think this is a solvable problem with a little more work.
- Readability. Right now, about one article out of four requires a little manual massaging. Paragraphs will be out of order, stray characters will occasionally appear, that sort of thing. Even with that, it only takes a minute or two to correct the flaws with the copy.
- No linking to other sources. I haven’t yet found a way to automatically include in-body hyperlinks to other related content on the internet, which would help make the articles more useful.
- Categorizing the posts is a manual process. For high-level categories, such as “U.S. News”, “World News”, “Sports”, etc., you want to have a limited number of categories to simplify navigation and discovery, and classifying the posts into the categories you have chosen to have is now a manual change. I have a strategy to correct this in a future iteration, however.
Correcting these problems will make the website almost autonomous, which is my near-term goal.
What kinds of other projects can News Mash be used for?
I currently have plans to try to adapt this system to some other kinds of web sites, including:
- Local/hyperlocal news reporting
- Special interest sites, such as gaming, movies, etc.
- Other uses I don’t yet wish to disclose.
Final thoughts
All things considered, I think this was a pretty educational and productive use of some free time. Constructing the python application and the associated web site took me less than a week to complete, and should continue generating new content for me for the foreseeable future. The era of everyday useful artificial intelligence has finally arrived, and I for one couldn’t be more pleased.
Got comments or questions? Feel free to send them my way using my contact form.